Welcome to the inaugural edition of the AI4AGRI newsletter!
We are thrilled to have you join us on this exciting journey as we explore the intersection of Earth observation, artificial intelligence and agriculture. Launched in October 2022, AI4Agri is dedicated to transforming the agricultural landscape through innovative AI solutions, enhancing productivity, sustainability, and resilience in the sector.
While our project has been underway for several months, we are launching this newsletter now to share the significant strides we’ve made and to keep you informed of ongoing developments. Our goal is to create a vibrant community of researchers, stakeholders, and technology enthusiasts who are passionate about leveraging AI to address the pressing challenges in agriculture using Earth observation and other data.
In this monthly newsletter, you’ll find insights into the AI4Agri activities, success stories from the field, publications, calls related to AI4Agri challenges, a focus on a scientist from the field, and expert opinions on the future of AI in agriculture. Thank you for joining us, and we look forward to embarking on this transformative journey together!In this issue, we highlight Mihai Ivanovici, leader of AI4Agri project. We present the AI4Agri school sheduled in May and the O3T Annual Meeting in Montpellier on June 17-18, 2024. We feature the publications from AI4Agri published in 2023.
Member Highlight
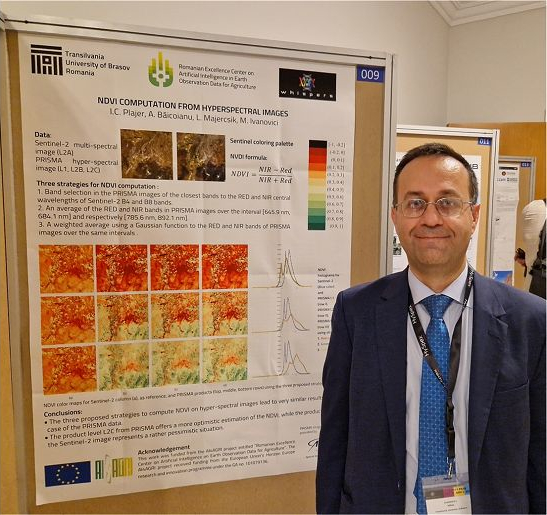
Mihai Ivanovici
Professor – UNITBV, Brasov (Romania)
Prof. Mihai Ivanovici holds a PhD in electronics from Politehnica University of Bucharest, Romania. He is a full professor and has 15 years of experience in managing various research projects (funded by EU structural funds and the Romanian government, the Ministry of Education and Research or by Romanian private companies) and participating as researcher in national and international research projects (e.g. The ATLAS Experiment at LHC). He is head of MIV Laboratory, within Department of Electronics and Computers,, Transilvania University of Brașov, România and member of the IEEE Signal Processing and IEEE Geoscience and Remote Sensing societies. He is a member of the Reviewers Board for MDPI Remote Sensing journal. His research interest and expertise are in the field of colour, multispectral and hyperspectral image processing and analysis. He is supervising PhDs in the field of electronics, telecommunications and information technologies. He is currently leading the EU funded AI4Agri project.
Call for Participation
AI4AGRI summer school – Artificial Intelligence for Earth Observation Data Analysis
📍 Transilvania University of Brașov
📅 Wed. 8 – Tue. 14 May 2024
http://ai4agri.unitbv.ro/wp-content/uploads/2024/01/AI4AGRI-Summer-School-2024-Call-for-participation.pdf
We are pleased to invite you to participate in the AI4AGRI Summer School 2024, taking place from May 8th to 14th in Brasov, Romania. This intensive program will focus on the cutting-edge applications of Artificial Intelligence (AI) and Earth Observation (EO) data analysis in agriculture. Participants will gain hands-on experience through a variety of modules, including hyperspectral image processing, semantic segmentation, and digital transformation in agriculture. Hosted by the R&D Institute of Transilvania University of Brasov, the summer school promises a comprehensive curriculum delivered by leading experts in the field. Accommodation will be provided at no cost to participants, fostering a collaborative and immersive learning environment. This is a unique opportunity for BSc, MSc, PhD students, and early-stage researchers to enhance their expertise and network with professionals from across the globe.
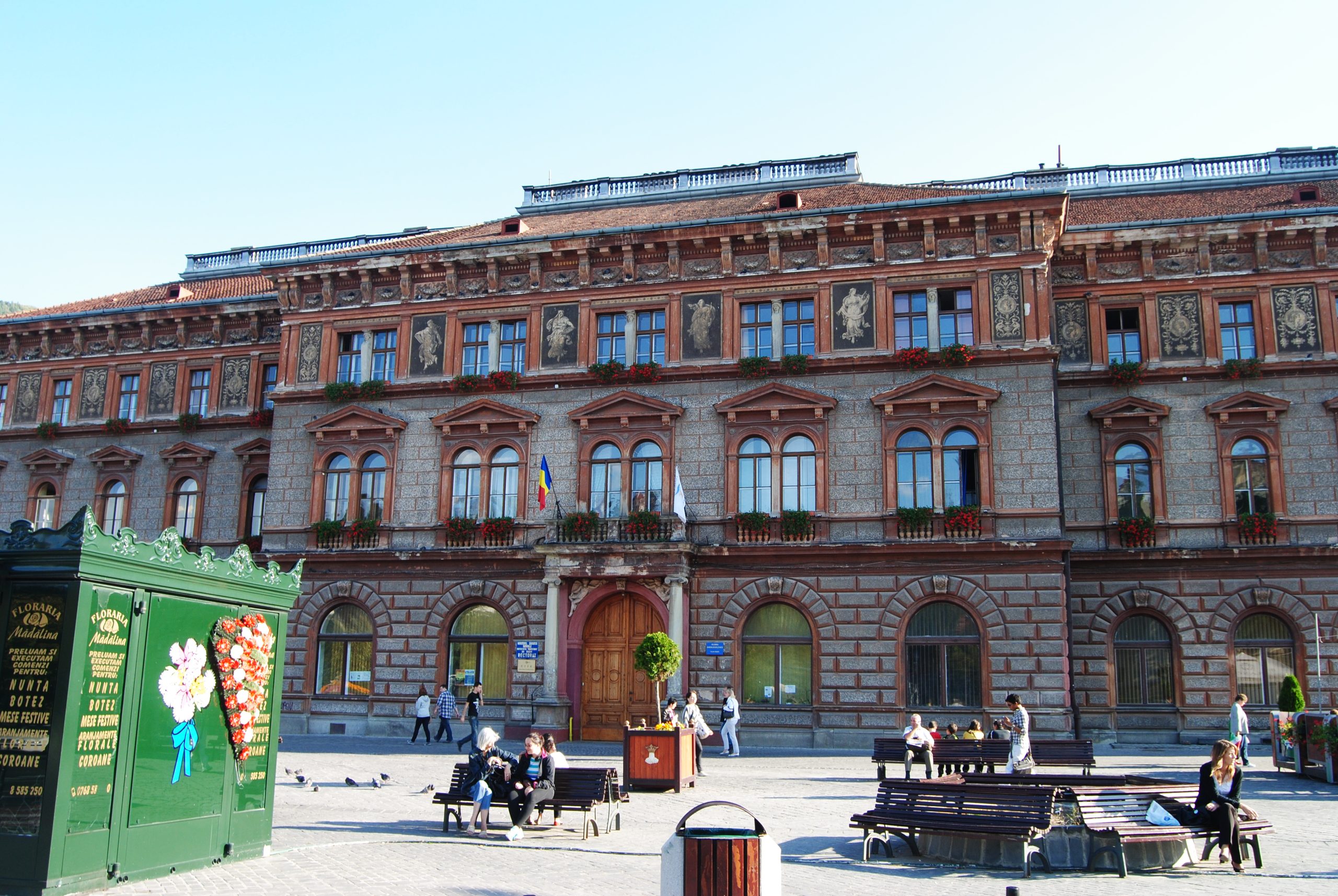
Upcoming Events
O3T Annual Meeting
📍 Maison de la Télédétection – Montpellier
📅 Mon. 17 – Tuesday 18 June 2024
https://inrae-fr.zoom.us/j/5788185359?pwd=QmNiTktRdWpaRjFmeWhKNitRNDZLUT09&omn=94986433947
Join us for the Annual O3T Seminar at the Maison de la Télédétection in Montpellier on June 17-18. The seminar will feature workshops, presentations, and discussions on urban and mountain environments, satellite reception, and various laboratory projects focused on transitions. Highlights include project presentations on climate change, biodiversity conservation, ecological and social transitions, and the application of AI in environmental monitoring. The event offers a platform for collaboration and innovation in addressing key regional and global challenges.
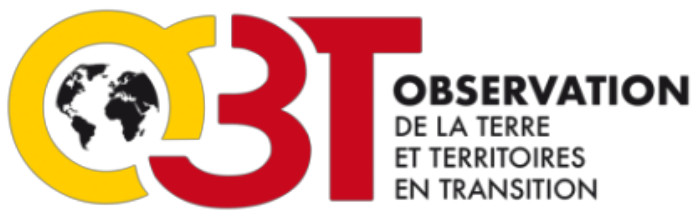
Upcoming meetings
AI4AGRI Monthly meeting
📅 Tue. 25 June – 14:00 (Fr time) | 15:00 pm (Ro time)
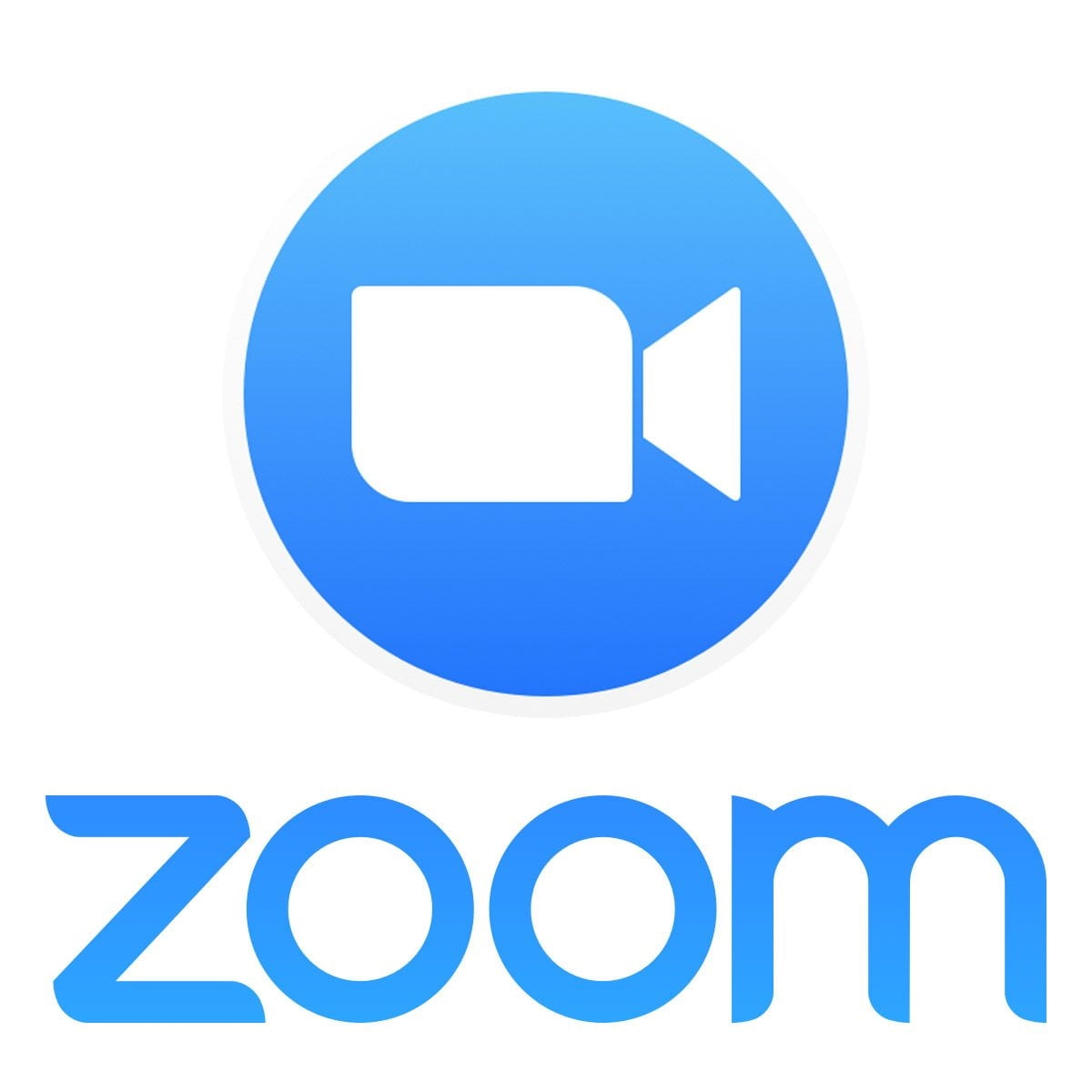
Latest AI4AGRI Publications
NDVI Computation from Hyperspectral Images (October 2023)
S. Oprisescu, R. M. Coliban, M. Ivanovici
#Visualization #Image color analysis #Vegetation mapping #Crops Sensors #Task analysis #Hyperspectral imaging
http://ai4agri.unitbv.ro/wp-content/uploads/2024/04/I_C_Plajer_WHISPERS_2023.pdf
In their recent research article, I. C. Plajer, A. Băicoianu, L. Majercsik, and M. Ivanovici delve into the application of multispectral and hyperspectral satellite imagery in modern agricultural practices. Their study focuses on the computation of vegetation indices, particularly the Normalized Difference Vegetation Index (NDVI), using data derived from hyperspectral images. The authors compare alternative methods for generating vegetation maps from these rich datasets, specifically contrasting NDVI maps produced from Sentinel-2 and PRISMA satellite data. Their comparative analysis highlights the nuances and distinctiveness between the two datasets, providing valuable insights into interpreting these indices for effective agricultural management strategies.
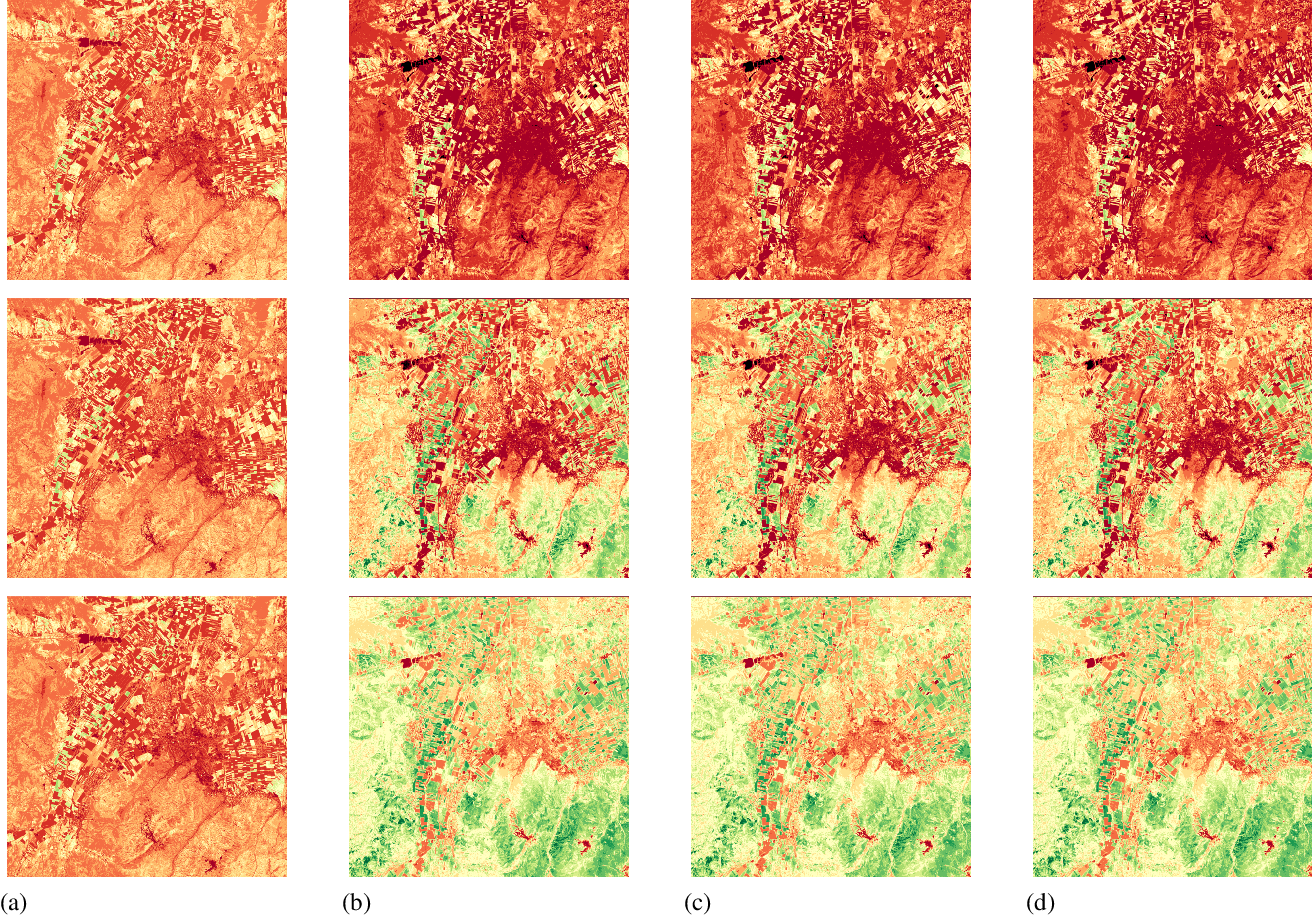
Multispectral Fractal Image Analysis for Soil Roughness Estimation at Various Altitudes (October 2023)
K. Marandskiy, M. Ivanovici, S. Corcodel and S. Costache
#Estimation #Soil #Gray-scale #Autonomous aerial vehicles #Fractals #Complexity theory #Remote sensing
https://ai4agri.unitbv.ro/wp-content/uploads/2024/04/K_Marandskiy_WHISPERS_2023.pdf
In their recent study, Marandskiy, Ivanovici, Corcodel, and Costache explore the use of convolutional neural networks for soil roughness estimation through the analysis of multi-spectral images. The research examines how fractal complexity, indicative of soil and vegetation properties, varies with sensor altitude in remote sensing scenarios. Utilizing an unmanned aerial vehicle, the authors assess the fractal dimension of images with five spectral bands, investigating the relationship between computed fractal complexity, altitude, and the number of spectral bands considered. This work provides valuable insights into the impact of image complexity on soil roughness estimation, with potential applications in agricultural monitoring and management.

Annotating Satellite Images of Forests with Keywords from a Specialized Corpus in the Context of Change Detection (September 2023)
N. Neptune and J. Mothe
http://ai4agri.unitbv.ro/wp-content/uploads/2024/04/N_Neptune_CBMI_2023_v1.pdf
Neptune and Mothe introduce a method for detecting deforestation in the Amazon rain forest using satellite image pairs. Their approach employs deep learning techniques to compare images captured at different times, effectively identifying changes in forest cover. A key innovation of their work is the integration of a visual semantic model that automatically annotates detected changes with pertinent keywords sourced from scientific literature on the Amazon region. The method is validated on a dataset of Amazon image pairs, demonstrating its capability to detect deforestation accurately while providing meaningful annotations. This research not only contributes to environmental monitoring efforts but also underscores its potential applicability across diverse domains beyond deforestation analysis.
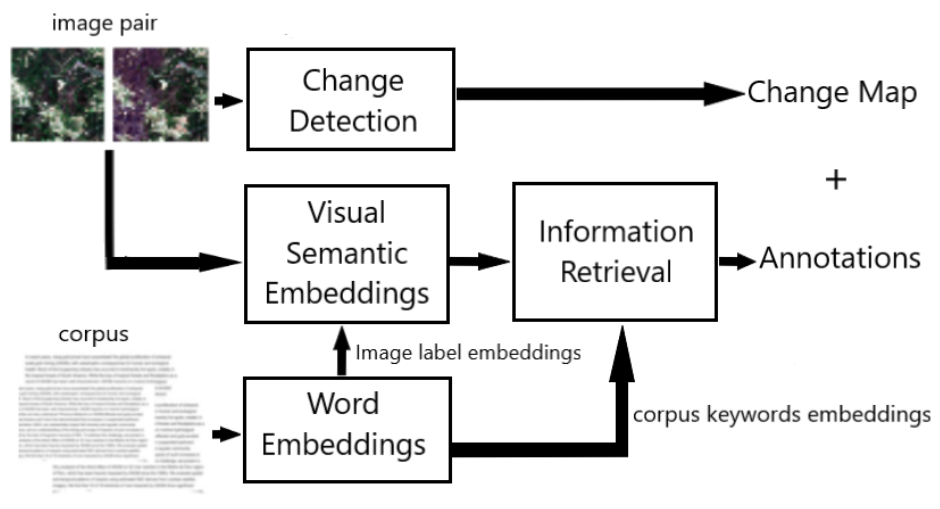
AI-Based Visualization of Remotely-Sensed Spectral Images (July 2023)
I. Plajer, A. Baicoianu, L. Majercsik
#Visualization #Interpolation #Satellites #Image color analysis #Military computing #Neural networks #Military satellites
http://ai4agri.unitbv.ro/wp-content/uploads/2024/04/I_C_Plajer_ISSCS_2023.pdf
Plajer, Baicoianu, and Majercsik present a novel approach to address the challenge of interpreting multispectral and hyperspectral satellite imagery in their recent study. Their work focuses on developing a robust artificial intelligence model for visualizing spectral images in the RGB color space. The proposed method utilizes a fully-connected neural network trained on the CAVE dataset, renowned for its diverse color representation in the visible spectrum. By applying this approach to a hyperspectral PRISMA image, the study aims to enable a visually consistent mapping essential for various applications, including agriculture, ecology, and defense. Initial results demonstrate promising outcomes, underscoring the potential of AI-driven techniques in enhancing the interpretation and utilization of remote sensing data.
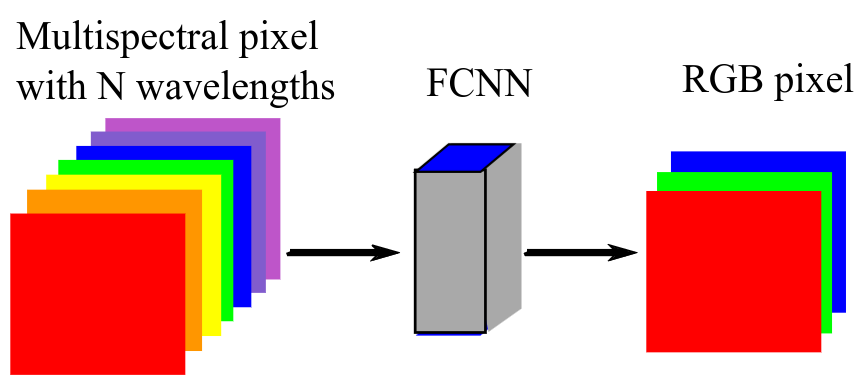
Hyperspectral Image Segmentation and Entropy Calculation for Qualitative Analysis of Grassland (July 2023)
S. Oprisescu, R. M. Coliban, M. Ivanovici
#Reflectivity #Image segmentation #Histograms #Thresholding (Imaging) #Image resolution #Entropy #Indexes
http://ai4agri.unitbv.ro/wp-content/uploads/2024/04/S_Oprisescu_isscs2023.pdf
The work presented by Oprisescu, Coliban, and Ivanovici focuses on the application of hyperspectral imaging for qualitative analysis of grassland areas. Hyperspectral imaging, with its capability to capture detailed spectral information across numerous bands, is increasingly valuable in Earth Observation tasks such as land cover mapping and agricultural assessments. In this study, the authors propose a semi-automatic approach to estimate the Shannon-Weaver biodiversity index using hyperspectral data. They initiate their analysis by selecting a representative spectral reflectance curve for grassland and subsequently perform image segmentation based on histogram thresholding of Spectral Angle Mapper (SAM) values. Following segmentation, entropy calculations are conducted on pixels identified as belonging to grassland areas. To facilitate entropy computation according to Shannon’s definition, a clustering step is employed for dimensionality reduction of the data. The effectiveness of the proposed methodology is assessed against manually established ground truth data, providing a structured and methodical framework for biodiversity assessment in grassland ecosystems using remote sensing techniques.
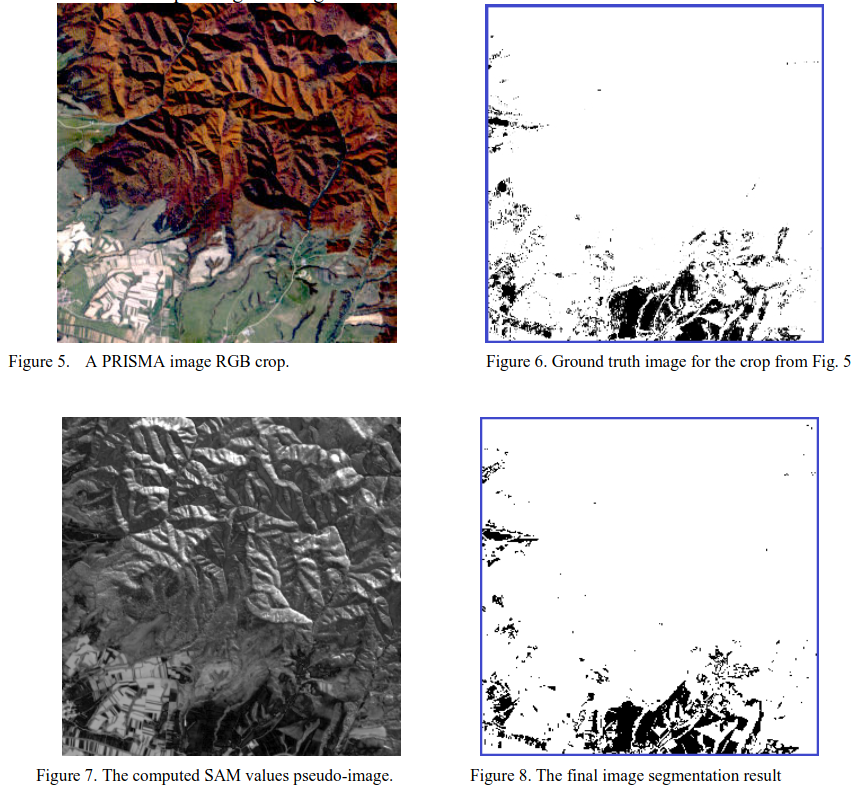
Soil Roughness Estimation Using Fractal Analysis on Digital Images of Soil Surface (July 2023)
I. C. Plajer, A. Băicoianu, L. Majercsik and M. Ivanovici
#Correlation #Soil measurements #Digital images #Estimation #Land surface #Soil #Surface roughness #Fractals #Rough surfaces #Surface texture
http://ai4agri.unitbv.ro/wp-content/uploads/2024/04/Kamal_ISSCS_2023.pdf
I. C. Plajer, A. Băicoianu, L. Majercsik, and M. Ivanovici addressed the crucial issue of soil surface roughness estimation, a parameter influenced by various agricultural practices and environmental factors. Traditional methods such as chain and pinboard techniques were initially explored both in laboratory and field conditions. However, discrepancies arose particularly in in-situ applications, prompting the researchers to propose a novel approach. Their innovative method employs fractal analysis of digital images captured using a camera obscura-based setup. This 2D fractal analysis was found to offer more relevant insights compared to conventional 1D methods, marking a significant advancement in soil roughness assessment techniques.
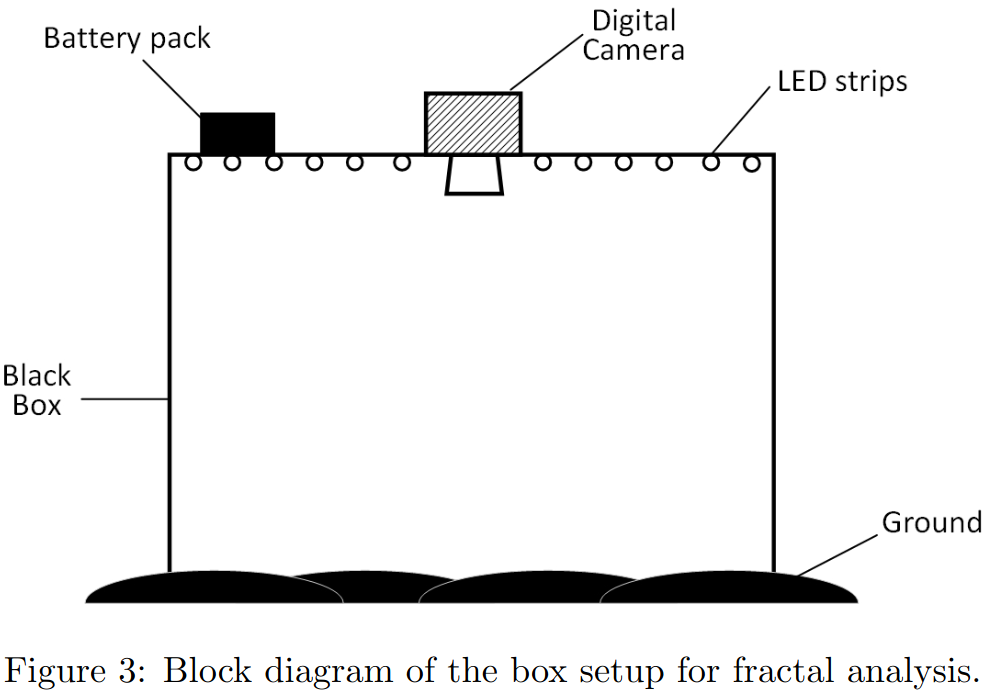
Convolutional neural network hardware implementation for soil roughness estimation (July 2023)
S. Popa, K. Marandskiy, G. Feldioreanu, M. Ivanovici
#VGG #convolutional neural network #soil roughness estimation
https://ai4agri.unitbv.ro/wp-content/uploads/2024/04/M_Ivanovici_abstract_EARSeL2023.pdf
In the context of Agriculture 5.0, researchers Stefan Popa, Kamal Marandskiy, George Feldioreanu, and Mihai Ivanovici have developed a hardware implementation of a Very Deep Convolutional Neural Network (VGG-11) for estimating soil surface roughness (SSR) from digital images. Utilizing the Python PyTorch framework, their model achieves 99.42% accuracy in estimating SSR’s Random Roughness (RR) parameter, which is crucial for understanding soil water storage, infiltration, and overland flow. This implementation, targeted at FPGA devices, aims to enable real-time, high-precision SSR estimation on portable or mobile platforms such as unmanned aerial vehicles. Their work serves as a validation model for further hardware development using Verilog.
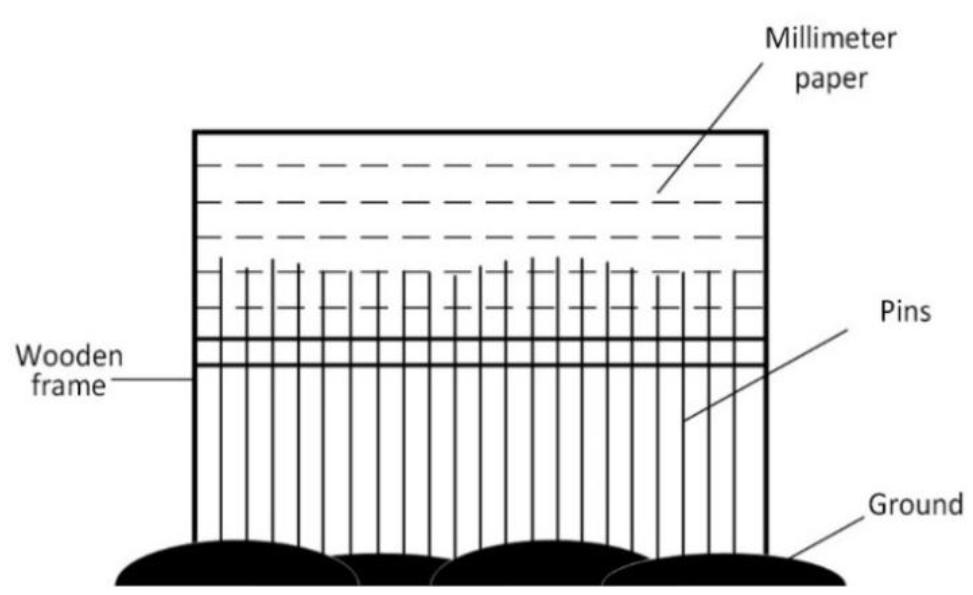
Exponential Feature Extraction and Learning for Pixel-Wise Hyperspectral Image Compression (July 2023)
M. Ivanovici, K. Marandskiy
#Image coding #Image color analysis #Measurement uncertainty #Data visualization #Machine learning #Feature extraction #Molecular biology
http://ai4agri.unitbv.ro/wp-content/uploads/2024/04/M_Ivanovici_IGARSS_2023.pdf
In their recent research article, I. C. Plajer, A. Băicoianu, L. Majercsik, and M. Ivanovici delve into the application of multispectral and hyperspectral satellite imagery in modern agricultural practices. Their study focuses on the computation of vegetation indices, particularly the Normalized Difference Vegetation Index (NDVI), using data derived from hyperspectral images. The authors compare alternative methods for generating vegetation maps from these rich datasets, specifically contrasting NDVI maps produced from Sentinel-2 and PRISMA satellite data. Their comparative analysis highlights the nuances and distinctiveness between the two datasets, providing valuable insights into interpreting these indices for effective agricultural management strategies.
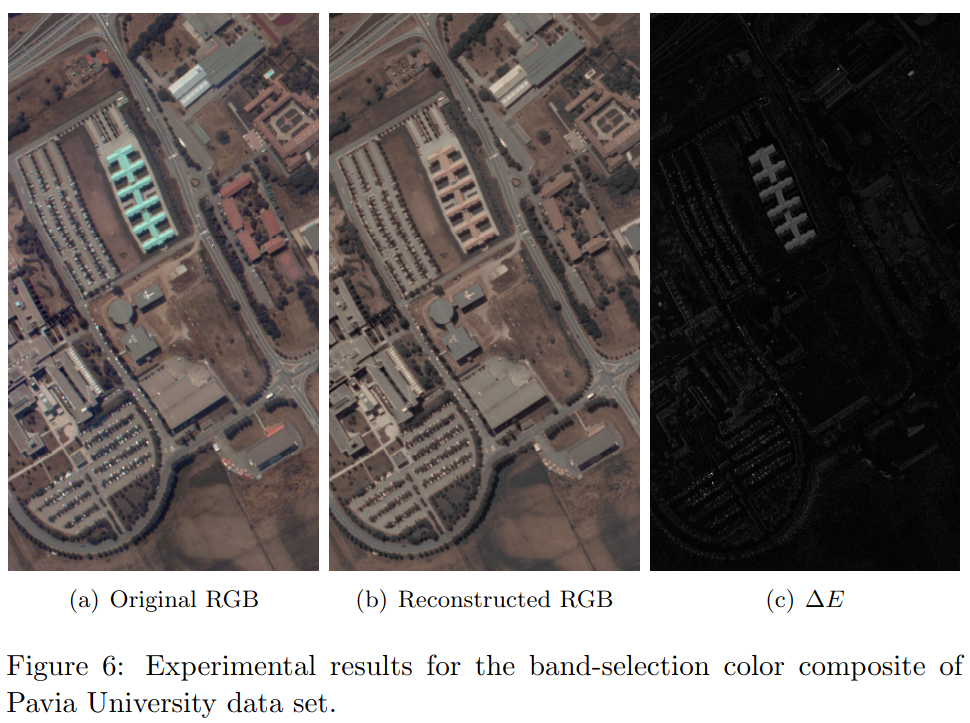
Exponential Features in the Fourier Domain for PRISMA Hyperspectral Image Segmentation (July 2023)
M. Ivanovici, S. Oprisescu, R.M. Coliban, K. Marandskiy
#Reflectivity #Earth #Image segmentation #Image resolution #Forestry #Feature extraction #Surface fitting
https://doi.org/10.1109/IGARSS52108.2023.10282239
The research conducted by M. Ivanovici, S. Oprisescu, R.M. Coliban, and K. Marandskiy focuses on advancing hyperspectral image segmentation techniques, crucial for applications in remote sensing such as precision agriculture and forest monitoring. Hyperspectral imaging provides detailed spectral information of Earth’s surface, but it also poses challenges due to the high variability in spectral reflectance curves, leading to increased data volumes. Addressing this, the study introduces a novel feature extraction method aimed at reducing dimensionality by modeling the Fourier spectrum of each spectral reflectance curve with a negative exponential function. This approach aims to mitigate the variability in spectral signatures, potentially enhancing segmentation accuracy. The researchers then implement a segmentation algorithm based on these extracted features, evaluating its effectiveness in practical applications.
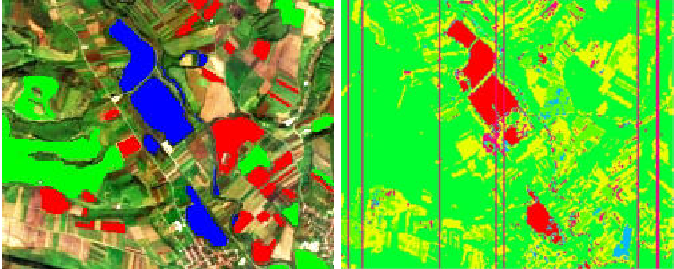
Hyperspectral Image Visualization Based on Maximum-Reflectance Wavelength Colorization (June 2023)
K. Marandskiy, M. Ivanovici,
#Reflectivity #Surface waves #Data visualization #Metals #Hyperspectral imaging
http://ai4agri.unitbv.ro/wp-content/uploads/2024/04/I_C_Plajer_WHISPERS_2023.pdf
In their recent research article, I. C. Plajer, A. Băicoianu, L. Majercsik, and M. Ivanovici delve into the application of multispectral and hyperspectral satellite imagery in modern agricultural practices. Their study focuses on the computation of vegetation indices, particularly the Normalized Difference Vegetation Index (NDVI), using data derived from hyperspectral images. The authors compare alternative methods for generating vegetation maps from these rich datasets, specifically contrasting NDVI maps produced from Sentinel-2 and PRISMA satellite data. Their comparative analysis highlights the nuances and distinctiveness between the two datasets, providing valuable insights into interpreting these indices for effective agricultural management strategies.
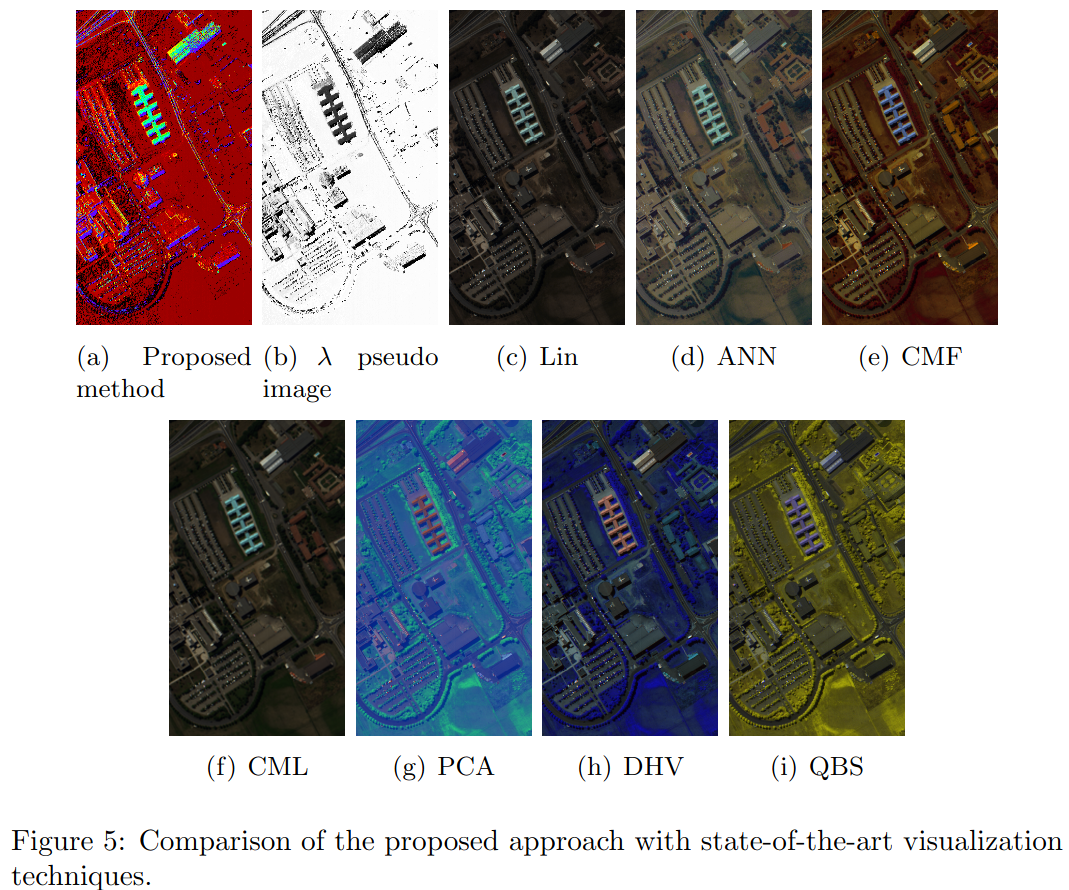
A Multi-Spectral Fractal Image Model and Its Associated Fractal Dimension Estimator (March 2023)
M. Ivanovici
#Fractal dimension #Box counting #Multi-spectral fractal images #Remotely sensed multi-spectral images
https://www.tandfonline.com/doi/full/10.1080/22797254.2024.2352386
Mihai Ivanovici presents a probabilistic fractal model and a fractal dimension estimator tailored for multi-spectral images. Building on the fractional Brownian motion fractal model, Ivanovici extends it to encompass images with multiple spectral bands, assuming statistical independence among these bands. The model is validated mathematically and used to generate synthetic multi-spectral fractal images with seven independent spectral bands in the visible domain. The associated fractal dimension estimator adapts the probabilistic box-counting approach for multi-spectral images. This methodology is tested on synthetic images and applied to real remotely sensed datasets, demonstrating its effectiveness in assessing image complexity.
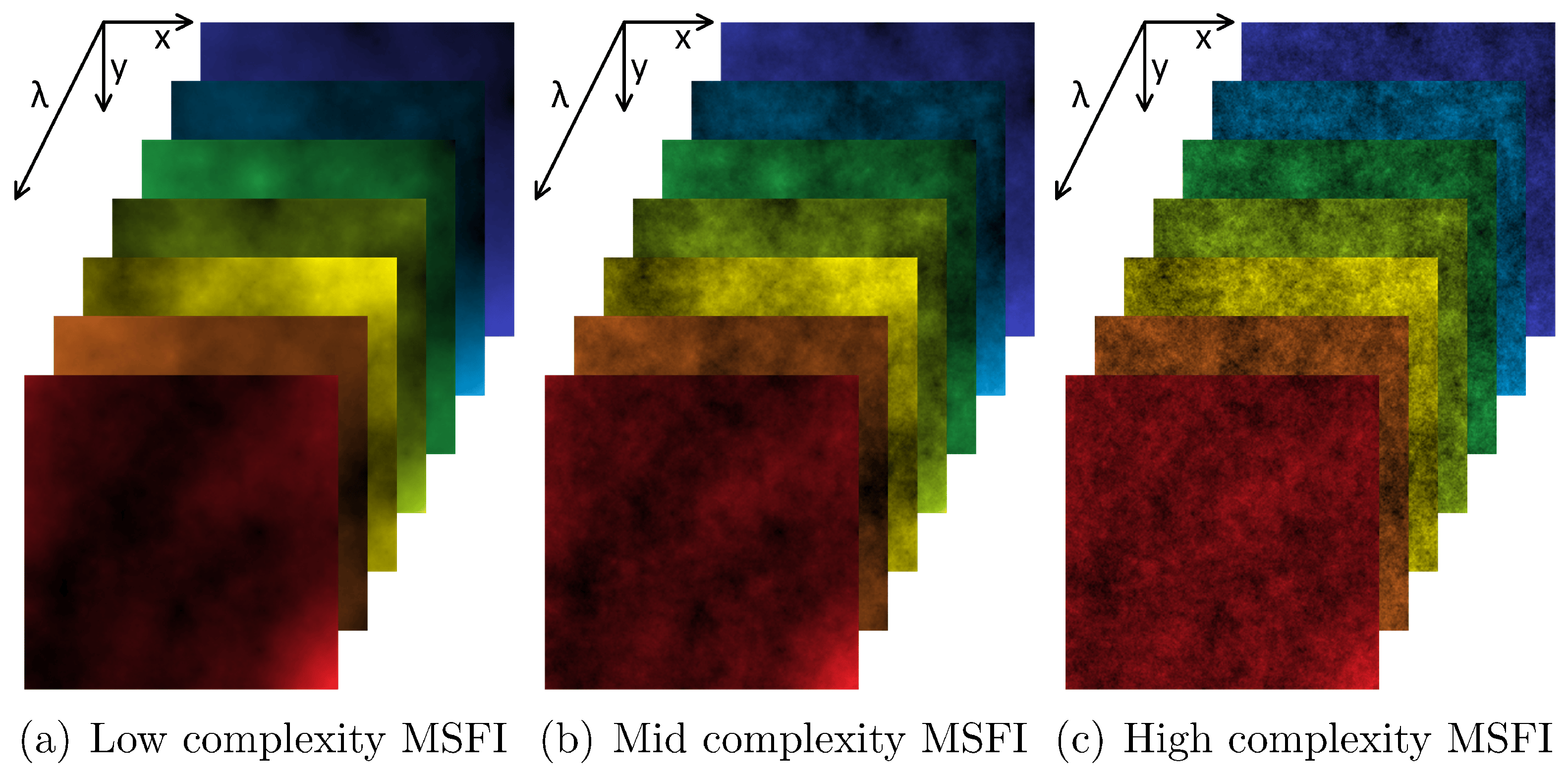
The AI4AGRI project received funding from the European Union’s Horizon Europe research and innovation programme under the grant agreement no. 101079136.
Publishing manager: J. Mothe & S. Molina, UT3 & UT2, IRIT, France