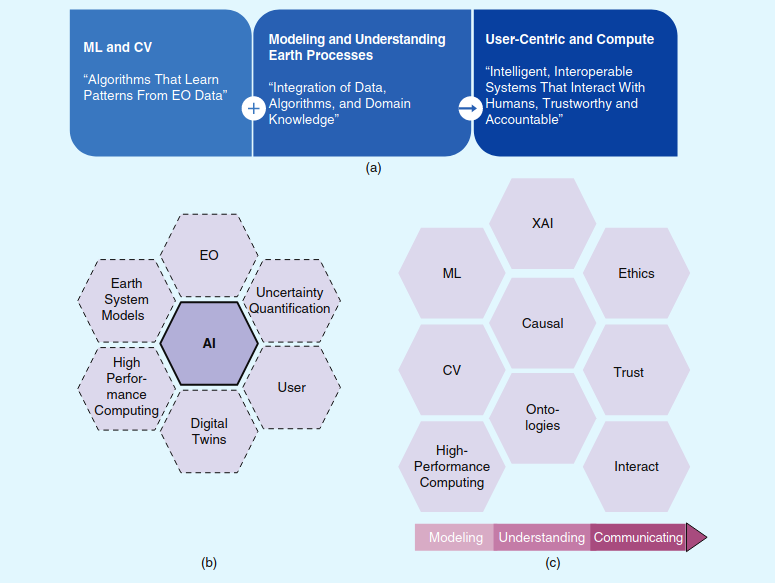
📅 September 2024
🧑 Devis Tuia, Konrad Schindler, Begüm Demir, Xiao Xiang Zhu, Mrinalini Kochupillai, Sašo Džeroski, Jan N. van Rijn, Holger H. Hoos, Fabio Del Frate, Mihai Datcu, Volker Markl, Bertrand Le Saux, Rochelle Schneider, Gustau Camps-Valls
This research work explores the integration of machine learning (ML) and artificial intelligence (AI) in the field of Earth observation (EO). As satellite data continues to grow, offering global insights into environmental processes, there is a pressing need for advanced processing techniques to convert raw data into actionable information. The paper discusses recent advances in ML and deep learning (DL) methodologies, which are increasingly used in EO image processing to provide meaningful insights into areas such as land use, vegetation dynamics, and disaster monitoring. Despite these advances, challenges remain, including data fusion, physical model integration, and the complexity of EO-specific tasks. The review offers a comprehensive overview of AI’s role in EO, highlights emerging trends like hybrid AI-physics models and explainable AI, and suggests potential future research pathways, emphasizing the transformative potential of AI in addressing global challenges like climate change and biodiversity monitoring.
https://ieeexplore.ieee.org/document/10669817
Tuia, D., Schindler, K., Demir, B., Zhu, X.X., Kochupillai, M., Džeroski, S., van Rijn, J.N., Hoos, H.H., Del Frate, F., Datcu, M. and Markl, V., 2024. Artificial Intelligence to Advance Earth Observation: A review of models, recent trends, and pathways forward. IEEE Geoscience and Remote Sensing Magazine.
@article{tuia2024artificial,
title={Artificial Intelligence to Advance Earth Observation: A review of models, recent trends, and pathways forward},
author={Tuia, Devis and Schindler, Konrad and Demir, Beg{\"u}m and Zhu, Xiao Xiang and Kochupillai, Mrinalini and D{\v{z}}eroski, Sa{\v{s}}o and van Rijn, Jan N and Hoos, Holger H and Del Frate, Fabio and Datcu, Mihai and others},
journal={IEEE Geoscience and Remote Sensing Magazine},
year={2024},
publisher={IEEE}
}